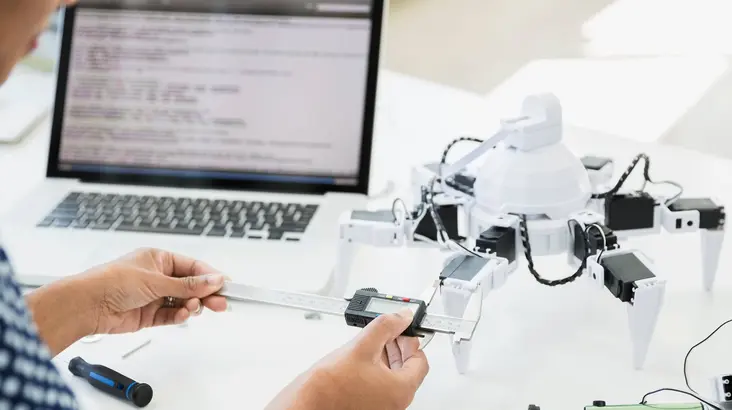
4 June 2020
AI and machine learning have long been touted as one of the ‘hottest trends’ in cybersecurity, not least by vendors enthusiastic to pitch defensive applications of the technology. Now we’re seeing AI edge closer to real-life application; but not in the way organisations would wish to see. Based on Etienne Greeff’s RSA speech, this blog makes the argument that AI & ML are fundamentally better suited to offensive applications than defensive – and this is a major problem. The blog will explain why this is the case, showing how training ML models is more applicable to the tasks being employed by attackers. It finishes with some practical advice for companies on what they can do now to defend against such attacks.
Debunking the myth
At Orange Cyberdefense, we regularly see enthusiastic marketing materials and press headlines launching new AI-based cyber-defense solution to combat attackers’. For the typical enterprise, this sounds great. Imagine being able to set up an intelligent security model and let it do all the work of identifying and resolving threats? I’d sign up for that right away!
The reality, however, isn’t quite so simple. Our team has thoroughly assessed the potential of AI in cybersecurity and we have arrived at a conclusion you probably won’t want to hear: AI is fundamentally better suited to offensive rather than defensive applications. That’s right, it’s going to help the bad guys do more, with less, and at a much greater scale. The good guys (that’s you) will need to keep up.
Machine Learning, AI and the Future of Cybersecurity
Firstly, to understand the application of AI in cybersecurity, it’s important to know how machine learning (ML) models learn. It is important to draw a distinction between ‘supervised’ and ‘unsupervised’ learning. It’s worth noting that in cybersecurity we typically do not have good data sets and this makes training models to detect targeted attacks difficultly, regardless of the training process.
What is The Difference Between Supervised and Unsupervised Learning?
Supervised learning is where data is labelled and used to teach ML models to predict the outcome of future data. In order for this to happen, new data needs to look the same as the data that has been used to train the models. The models are instantly able to recognise and process the data and act upon it. Self-driving cars are an example of supervised learning most people would be familiar with.
Unsupervised learning is more complex. It’s where there is lots of data but no labels. The system has no comprehension of what the data is but is trying to find commonalities in order to understand the structure of data and detect patterns. Such models have no way of detecting abnormal or outright suspicious data.
This distinction between supervised and unsupervised can help us to understand the inbalance in how AI can serve the purposes of attackers and of enterprises.
Cybersecurity With Artificial Intelligance
Enterprises face attacks from all vectors. The nature of the attacks is continually evolving and attackers are finding new and novel tactics to penetrate networks. Applying AI here won’t necessarily lead to better outcomes as it is extremely difficult to train AI models to detect threats and abnormalities that the system doesn’t already recognise. In essence, the data required is unstructured and so it’s difficult for the models to act upon it.
For the attackers, the situation is different. They need highly repeatable models that allow them to carry out the same attacks time and time again on different networks. Not every attack will be successful, but the more attempts they make, the increased probability of effective penetration of a network. It’s a numbers game.
AI supports this proposition well. Attackers can use supervised learning models to allow them to repeat attacks at scale and therefore increase their chances of winning. While they are still in their nascent stages, the types of AI-based attacks we expect to see include ‘DeepPhishing’ (using deep learning to bypass AI-based phishing detection), fooling deep learning-based image recognition, web application attacks, and bug hunting in libraries. These are all based on tactics attackers use currently, but applying AI means they can automate and operate at a greater scale.
These types of attacks will likely become more common as attackers seek to exploit flaws in your network, make broad sense of the data you hold and focus in on what has specific value.
Getting prepared
So what can your enterprise do to prepare for a (not-so-far-off) future where the attackers are wielding better weapons than you? There are a few points of advice Orange Cyberdefense would offer.
Firstly, don’t assume that the risk of offensive AI is remote. The models are already seeing practical application and will move towards the mainsteam sooner not later. As a business you need to be ready. Readiness involves understanding the new threat models that AI & ML may introduce, and being able to see how an attacker would use them in your network.
Furthermore, we would advise that you test the robustness of your environment in the way an attacker would. In order to spot feature-based attack opportunities you need to understand where data lives and how an attacker might see it. Fortunately, there are a number of open source tools you can utilise to model such attacks on your own network in order to see your environment from a different perspective.
Finally, and perhaps most importantly, have a response plan ready. Offensive applications of ML are very plausible and possible, so you want to be ready for whatever your environment is faced with. Don’t try and wing it against intelligent machines.
To find out more about how Orange Cyberdefense can help you defend against offensive AI…contact us or call us on 01622 723400 and one of our team will be happy to help.